Migrating to NoSQL – MongoDB
Data Shards
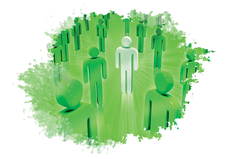
MongoDB combines the usual advantages of NoSQL databases with sharding, load balancing, replication, and failover.
Many products describe themselves with the NoSQL label. MongoDB [1] is arguably the most popular of these because it has seen incredible developer adoption. The NoSQL concept has advanced through the technology hype cycle [2], including wild claims about scalability [3], and MongoDB is making its way up the slope of enlightenment, having dealt with the trough of fear, uncertainty, and doubt [4]. With the help of 10gen, the MongoDB company, the product is now well on its way to addressing the enterprise market; however, what does that mean for you? Why should you migrate to MongoDB, and how should you do so?
After having switched an in-production app from MySQL to MongoDB back in early 2009, before even version 1.0 of MongoDB had been released, my company database now processes more than 20TB of data each month. With this experience under my belt, I'm going to cover several important areas that should help you understand when to consider switching to MongoDB.
Client Libraries
Like MySQL, Postgres, or most other databases, MongoDB is language agnostic and provides a custom wire protocol that you use to talk to the database. Some NoSQL databases operate over plain HTTP, so although having a wire protocol means using a custom driver [5], it does offer advantages, such as lower overhead and a query language implementation that makes sense to whatever programming language you're working with.
Like most databases, MongoDB is open source, and with a clear spec, anyone can create their own client. The community drivers, I have found, were not well tested at high throughput, and with little or no support, you either have to fix bugs yourself or rely on author availability. However, the official 10gen drivers are well maintained and tested. This becomes particularly important once your project goes into production: You know you're dealing with a stable driver, and any issues are likely to be fixed in a timely manner. These official MongoDB drivers [6] are available for a wide range of languages, including C, C++, C#, Erlang, Java, JavaScript, Node.js, Perl, PHP, Python, and Ruby (Figure 1).
Schema Freedom
A big advantage of NoSQL databases is that users are not required to define a schema up front. Making structural changes with MySQL and other relational databases can require a great deal of effort, but documents in MongoDB are simply JSON files that can be changed on the fly and include structures like arrays and timestamps, or even other documents [7].
However, just because you can add fields at will doesn't mean you shouldn't think about document design at the beginning, especially if it leads to an increase in document size, threatening performance problems. For example, if you add new fields or if the size of your document (&0x2245; field names+field values) grows past the allocated space, your document will have to be rewritten elsewhere in the data file, creating a performance hit. If this happens a lot, Mongo will adjust paddingFactor
[8], so documents are allocated more space by default; in-place updates are faster.
One way to avoid rewriting an entire document and modifying documents in place is to specify only those fields you want to change and use modifiers where possible. Instead of sending a whole new document to update an existing one, you can set or remove specific fields.
The following query in the mongo
shell (Figure 2) updates the cats
field to 5
and sets the hats
field to 2
:
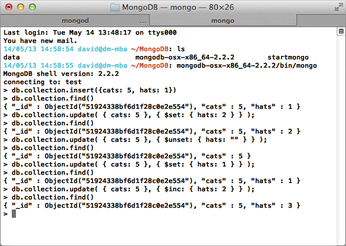
db.collection.update( { cats: 5 }, { $set: { hats: 2 } } );
The next query does the same thing, but instead of setting one of the fields, it removes it from the document:
db.collection.update( { cats: 5 }, { $unset: { hats: "" } } );
For certain operations, like incrementing, you can use modifiers. Instead of setting the hats
field to 2
as before, you can increment it by a certain number. Assuming its current value is 1
, the following query increments the field by 2
, yielding a final value of 3
.
db.collection.update( { cats: 5 }, { $inc: { hats: 2 } } );
These operations are more efficient in communicating with the database, as well as modifying the file.
Because a document might be rewritten just by changing a field data type, consider the format in which you want to store your data. For example, if you rewrite (float)0.0
to (int)0
, you are changing the BSON data type; therefore, familiarize yourself with the BSON specification [9].
Failover and Redundancy
Server Density, my company's product, handles a huge amount of data from monitoring customer servers and websites. Billions of metrics are recorded, and we need to ensure uptime and reliability, so we can alert customers when problems occur. This means replication across data centers, which was one of the biggest difficulties I had with MySQL – getting replication up and running and automating failover.
MongoDB uses the concept of replica sets, essentially a master/slave setup requiring a minimum of three nodes, any of which can become the master (by default). The instances communicate constantly via a heartbeat protocol, and if a failure is detected, one of the nodes is elected as master. This process all happens internally and is then picked up by the client drivers: An exception will be raised, and a reconnect happens automatically, so you can decide whether to show an error to the user or just retry silently.
This approach means only a minimal amount of work achieves redundancy with multiple copies of your database and automatic failover. Further options [10] allow you to:
- Define priorities to determine which nodes should become master, and in which order;
- Hide nodes, so they can store copies of the data, but never become master;
- Add and remove nodes with minimal or no effect on the replica set;
- Have some members deliberately stay behind the master by a certain amount of time (e.g., to allow recovery in the case of a catastrophic event, such as deleting a whole database); and
- Set up tiny nodes that help elect new masters but don't store any data (e.g., to make up the majority and help decide what happens in the event of a network split).
Consistency
In a master/slave setup, the slave always stays up to date with the master. Scaling of read operations occurs with the use of setSlaveOk
, causing the client library to distribute the reads automatically to the closest replica slave (on the basis of ping latency). In production, this will depend on a number of factors, including network latency and query throughput, which means MongoDB eventually becomes consistent, although the slaves might differ occasionally from the master.
To mitigate this problem, use write concern (Table 1) as part of the driver options when issuing writes. This value defaults to 1
, which means the call will not return until the write has been acknowledged by the server.
Table 1
Write Concerns
Write Concern | Meaning |
---|---|
|
Disables all acknowledgment and error reporting. |
|
Disables all acknowledgment, but will report network errors. |
|
Acknowledges the write has been accepted. |
n > |
Provides acknowledgment the write has reached the specified number of replica set members. |
|
Provides acknowledgment the write has reached the majority of replica set members. |
Tag name |
A self-defined rule (see text). |
For real replication and consistency, you can require acknowledgment by n slaves. At this point, the data has been replicated and is consistent across the cluster. The write concern can be a simple integer, the keyword majority
, or a self-defined tag.
Each step up the consistency ladder has an effect on performance [11] because of the additional time required to verify and replicate the write.
Be advised that an acknowledgment is not the same as a successful write – it simply acknowledges that the server accepted the write job. The next step is to require a successful write to the local journal. All writes hit the MongoDB journal before being flushed to the data files on disk, so once in the journal, the write is pretty much safe on that single node.
In this way, different levels of reliability are ensured. A high-throughput logging application would be able to tolerate losing a few write operations because of network issues, but user-defined alert configurations, for example, would need a higher level of reliability.
Tag Flexibility
To make your setup even more flexible, tags can remove replica set consistency from your code. Instead of specifying a number in the client call, you can define tags and then change them at the database level.
For example, in Listing 1, I have defined a replica set with two nodes in New York, two nodes in San Francisco, and one node in the cloud. I have also defined two different write modes: veryImportant
, which ensures data is written to a node in all three data centers, and sortOfImportant
, which writes to nodes in two data centers.
Listing 1
Replica Set Configuration
On the basis of this configuration, the following statements:
db.foo.insert({x:1}) db.runCommand({getLastError : 1, w : "veryImportant"})
write to one node in New York, one node in San Francisco, and one node in the cloud.
Sharding
Even with a fast network, plenty of RAM, and SSDs [11], you will eventually hit the limits of a single node within a replica set. At this point, you need to scale horizontally, which means sharding.
Sharding in MongoDB takes place without the need to modify your application. Instead of connecting directly to the database, you point your clients to a router process called mongos, which knows how the data is distributed across shards by storing metadata in three config servers.
Sharding sits on top of replica sets, so you have multiple nodes within a replica set, all containing copies of the same data. Each shard consists of one replica set, and data is distributed evenly among the shards.
MongoDB moves chunks of data around to ensure balance is maintained, but it's up to the admin to decide the basis on which those chunks are moved. This is known as your shard key and should allow you to split the data while avoiding hotspots. For example, if your shard key is a timestamp, data is split chronologically across the shards. This becomes a problem if you access data that's all in the same shard (e.g., today's date). Instead, a better key is something more random and not dictated by use patterns. The MongoDB documentation provides a more detailed look at this [12].
Sharding lets you add new shards to the cluster transparently, and data then moves to them. As your data grows, you simply add more shards. This method works if you maintain good operational practices, such as adding new shards before reaching capacity (because moving lots of documents can be quite an intensive operation). For example, if you increase the number of shards from two to three, 33% of your data will be moved to the new shard.
Conclusion
All NoSQL databases operate on similar principles: Lots of RAM and fast disks usually enhance performance; however, you should pay attention to the differences and tradeoffs, as you would any new technology.
Given MongoDB's popularity and widespread use, a great deal of knowledge has been shared online, in mailing lists, and at conferences. As a result, MongoDB use cases encompass everything from general-purpose to high-performance databases.
Infos
- 10gen website: http://www.10gen.com/leading-nosql-database
- Hype cycle: https://en.wikipedia.org/wiki/Hype_cycle
- MongoDB is web scale video: http://mongodb-is-web-scale.com/
- MongoDB FUD: http://blog.serverdensity.com/does-everyone-hate-mongodb/
- HTTP REST API: http://docs.mongodb.org/ecosystem/tools/http-interfaces/
- Drivers and client libraries: http://docs.mongodb.org/ecosystem/drivers/
- Data modeling: http://docs.mongodb.org/manual/core/data-modeling/
paddingFactor
implications: http://tebros.com/2010/11/mongodb-paddingfactor-implications/- BSON spec: http://bsonspec.org
- Replication: http://docs.mongodb.org/manual/replication/
- MongoDB benchmarks: https://blog.serverdensity.com/mongodb-benchmarks/
- MongoDB Manual 2.4.4: http://docs.mongodb.org/manual/core/sharded-cluster-internals/