Filtering log messages with Splunk
Needle in a Haystack
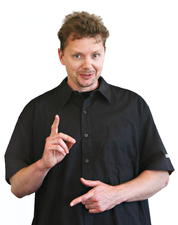
Splunk has mastered the art of finding truly relevant messages in huge amounts of log data. Perlmeister Mike Schilli throws his system messages at the feet of a proprietary analysis tool and teaches the free version an enterprise feature.
To analyze massive amounts of log data from very different sources, you need a correspondingly powerful tool. It needs to bring together text messages from web and application servers, network routers, and other systems, while also supporting fast indexing and querying.
The commercial Splunk tool [1] has demonstrated its skills in this field even in the data centers of large Internet companies, but the basic version is freely available for home use on standard Linux platforms. After the installation, splunk start
launches the daemon and the web interface, where users can configure the system and dispatch queries, as on an Internet search engine (Figure 1).
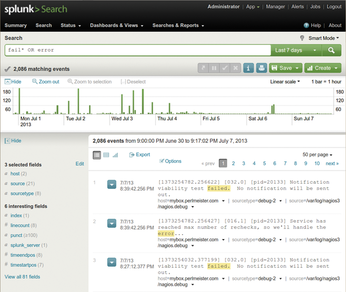
[...]