Big Data search engine for full-text strings and photos with radius search
Elastic Hits
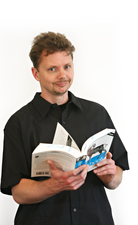
The Elasticsearch full-text search engine quickly finds expressions even in huge text collections. With a few tricks, you can even locate photos that have been shot in the vicinity of a reference image.
While I was looking for a search engine the other day that would crawl logs quickly, I came across Elasticsearch [1], an Apache Lucene-based full-text search engine that includes all sorts of extra goodies.
On the download page, the open source project offers the usual tarball and a Debian package. The 1.0.0.RC2 pre-release version, which was the latest when this issue went to press, can be installed without any problems on Ubuntu by typing sudo dpkg --install *.deb
. The Debian package includes a convenient boot script. When called by root at the command line as follows,
"#" /etc/init.d/elasticsearch start
the script fires up the Elasticsearch server on the default port of 9200.
Polyglot or Perl
Most hands-on Elasticsearch tutorials off the web use the REST interface to communicate with the server via HTTP. Figure 1 shows a GET
request on the running server, which displays its status.
Several REST clients in multiple languages can be used to feed in data and query it later. The CPAN Elasticsearch module is the official Perl client. Note, however, that Elasticsearch (current version 1.03) [2] is the successor to the obsolete ElasticSearch module (with an uppercase S) [3]. This was an unfortunate choice of name by the CPAN author, if only because the old version still resides on CPAN and pops up before the new one when you search on search.cpan.org.
As a useful sample application for an Elasticsearch full-text search, I chose a keyword search of all Perl columns previously published in Linux Magazine. The manuscripts of more than 130 articles in this series can be found in a Git repository below my home directory; the script in Listing 1 [4] sends all the recursively found text files via the REST interface to the running Elasticsearch server for indexing. The command
"$" fs-index ~/git/articles
took just a few minutes. A second call, with the disk cache warmed up, whizzed by in just 30 seconds. A subsequent search for the seemingly unlikely word "balcony" then returns results within a fraction of a second:
"$" fs-search balcony /home/mschilli/git/articles/water/t.pnd /home/mschilli/git/articles/gimp/t.pnd
The files found in the index reveal that I have only used the word "balcony" in two issues thus far: once in August 2008 in an article about a Perl interface for the GIMP image editor, in which I manipulated a photo shot from my own balcony [5]; and in April 2007, when I described an automatic irrigation system for my balcony plants [6].
Listing 1
fs-index
Fuzzy Search
Elasticsearch is not case-sensitive and handles stemming without any help. However, the indexer does not realize that "balconies" is the plural of "balcony" and provides no results in this case. Unfortunately, Elasticsearch sometimes takes things a little too far with the fuzzy search and presents matches that are not real matches, because the words start with the same string. Apart from that, the search function finds a needle in a haystack – and quickly.
Line 10 of the fs-index
script in Listing 1 accepts the search directory handed over to the script at the command line; it then calls the Elasticsearch
class constructor. If the search queries do not return the desired results and you wonder why, you can twist the constructor's arm:
my $es = Elasticsearch->new( trace_to => ['File','log'] );
It will then output all the commands sent to the Elasticsearch server in Curl format in the log
file. Using cut and paste, puzzled developers can then begin to gradually understand what's going on under the hood.
Elasticsearch stores the text data in an index, which is named fs
in this example (as in "filesystem") in line 8. If the index already exists, the delete()
method deletes it in line 17. The surrounding eval
block tacitly fields any errors – for example, if the index does not exist yet because this is the very first call to fs-index
.
Heavyweights and Binaries Excluded
The find()
function from the File::Find module starts to dig through the directories on the hard disk as of line 21, starting with the base directory passed in at the command line. Line 25 ignores any binary files, and anything that is not a real file or is larger than 100,000 bytes also is left out with additional tests. The slurp()
function from the CPAN Sysadm::Install module then sends the content of any files worth keeping to memory, which the index()
method in line 30 feeds to the database under the content
keyword. The name of the file also ends up there under keyword file
.
Mini-Google with Many Formats
Later, the script in Listing 2 finds files for predefined keywords, just like an Internet search engine. When you call this script with fs-search '*'
, the command matches every document in the index. (The single quotes stop the Unix shell from grabbing the metacharacter *
and turning it into a glob in the local directory.) Anyway, Elasticsearch returns 10 more or less random results for fs-search '*'
, because with no further configuration, the maximum number of hits is set to 10. The script shown a little later changes this value to 100.
Listing 2
fs-search
The search()
method called in line 12 expects the name of the search index under which the data resides (again fs
), and it wants the query string in the body
part of the request. From the documentation [7], you can see that Elasticsearch obviously understands a whole range of historically grown query formats, which necessitates the seemingly absurd nesting (lines 15-17) of query/query_string/query
.
The result of the fact-finding mission is a reference to an array of hits, which the for loop iterates over in lines 20 and 21, printing only the names of the matching files, which are part of the result set.
Searching GPS Image Data
Elasticsearch can do even more, though. For example, the geo_distance
filter [8] extends the classic full-text search, adding an interesting capability. If you configure the filter and store matching geodetic data for each document, the search engine will show you the entries that are located within a certain radius. This feature could be useful, for example, if you find yourself roaming around with your mobile phone late at night looking for a five-star restaurant in the area that is still serving.
Because my iPhone 5, just like any other smartphone, stores the GPS data in the Exif header of the JPEG file for every image I shoot, a search that starts with a given image in the phone's photo album ("Gallery") and finds images that I shot within a 1km radius of the image's location could be fun. As an example, Figure 2 shows a photo of the newly constructed eastern span of the Bay Bridge [9] in my city of residence, San Francisco. Since last year, pedestrians and bike riders can get to the middle of the bridge on a dedicated path, from where I shot a number of photos.
Figure 3 shows the output of the exiftags
command for the photos transmitted from the phone to my Linux machine. Almost at the bottom, you can see that the image was shot at the geolocation 37°48.87' north latitude and 122°21.55' west longitude.
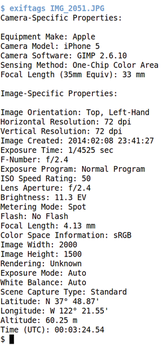
Modern Sextant
The photo_latlon()
function in Listing 3 reads these GPS values with the CPAN Image::EXIF module and uses dm2decimal()
from the Geo::Coordinates::DecimalDegrees module to convert them to floating point numbers. The regular expression in lines 47-53 searches the geodata for a letter (N or S for north or south latitude, W or E for western or eastern longitude), followed by the numerical degree value and the degree symbol encoded in UTF-8. The minutes follow after one or more spaces.
Listing 3
IPhonePicGeo.pm
Thus, 37°48.87'N becomes the value 37.816
and 122°21.55'W becomes the negative floating-point number -122.3555
.
Google Maps confirmed (Figure 4) that the talented photographer really was standing in the middle of San Francisco Bay on the Bay Bridge when he pressed the button. To find out whether other pictures in the photo album were recorded within a radius of 1km, photo-index
in Listing 4 examines all the transferred photos in the ~/iphone
directory and stores their GPS data on the local Elasticsearch server.
Listing 4
photo-index
Nice Scenery
The find()
function also recursively digs through subdirectories. For the search engine to store the geodata in a way that optimizes the query performance, I need to add a mappings directive: The create()
command as of line 15 defines a geo_point
property by the name of Location
for the photo
document type used in the photos
index. The documentation for this [8] is out of date, by the way; the mapping it describes no longer works. I have, however, successfully tested Listing 4 with Elasticsearch release 1.0.0 RC2.
Starting with the jpeg images found by the search, line 32 in Listing 4 uses the IPhonePicGeo module to extract the geodata and pushes it, along with the file names, into the elastic database in the body
section of the index()
method starting in line 35.
After the data of all the photos has been indexed in this way, the script in Listing 5 retrieves all the snapshots that I took within 1km of the reference photo passed in at the command line. For this purpose, it ascertains the geodetic information of the reference image and then sends a match_all()
query, which returns all stored images. Line 23 turns on a filter that limits the geo_distance
to 1km. Additionally, the size
parameter increases the maximum number of hits to 100
.
Listing 5
photo-gps-match
This returns a list of photo objects, of which line 37 extracts the original file name and pushes it to the end of the array @files
. Finally, the system()
function in line 40 calls eog
(The Eye of Gnome application), which displays all the results as thumbnails (Figure 5). You can now click your way through them to explore the vicinity.
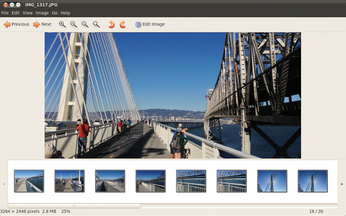
No Limits
The geo-function is just one of many plugin-like extensions of the Elasticsearch server, a useful tool that is easy to install and operate. It also scales practically infinitely because, as the volume of data increases, the administrator can distribute the indexes to a sufficiently large number of other Apache Lucene shards, to again run all queries with the required level of performance.
Books on paper and electronic form exist for Elasticsearch, but unfortunately, I can't really recommend any of them. That said, however, the tutorial [10] can be a help, and volunteers will answer questions on Stackoverflow.com.
Mike Schilli
Mike Schilli works as a software engineer with Yahoo! in Sunnyvale, California. He can be contacted at mschilli@perlmeister.com. Mike's homepage can be found at http://perlmeister.com.
Infos
- Elasticsearch download site: http://www.elasticsearch.org/overview/elkdownloads/
- Elasticsearch-1.03: http://search.cpan.org/~drtech/Elasticsearch-1.03/
- ElasticSearch-0.66: http://search.cpan.org/~drtech/ElasticSearch-0.66/
- Listings for this article: ftp://ftp.linux-magazin.com/pub/listings/magazine/162
- "Card Trick" by Mike Schilli: http://www.linux-magazine.com/w3/issue/95/072-076_perl.pdf
- "Don't Blame the Gardener" by Mike Schilli: http://w3.linux-magazine.com/issue/77/Perl_Linux-based_Gardening.pdf
- Elasticsearch documentation: http://www.elasticsearch.org/resources/
- Elasticsearch geo--distance filter: http://www.elasticsearch.org/guide/en/elasticsearch/reference/current/query-dsl-geo-distance-filter.html
- "New Bay Bridge spanning San Francisco Bay finally finished" by Mike Schilli: http://usarundbrief.com/103/index-en.html
- Elasticsearch tutorial: http://joelabrahamsson.com/elasticsearch-101/