Python network data visualization
Data Harvest
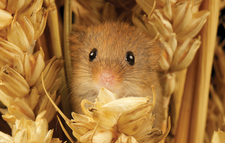
© Lead Image © Mark Bridger, 123RF.com
The Scapy packet manipulation program lets you analyze and manipulate packets to create incident response reports or examine network security.
Most folks have pulled up Wireshark a time or two to troubleshoot an application or system problem. During forensics, packet captures (PCAPs) are essential. Often you are looking at things like top talkers, ports, bytes, DNS lookups, and so on. Why not automate this process with Python?
Scapy [1] is a great tool suite for packet analysis and manipulation. It is most often talked about in the realm of packet manipulation, but its ability to analyze packets is also top-notch.
Make Ready
First, you need to make sure you have Python 3 installed along with the following packages:
[...]