Looking for an edge with the classic Quicksort algorithm
Smart Sort
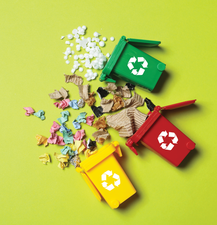
© Lead Image © Olga Yastremska, 123RF.com
If you wanted to assign a flavor to the Quicksort algorithm, it would be sweet and sour. Sweet, because it is very elegant; sour, because typical implementations sometimes leave more questions than they answer.
The Quicksort sorting algorithm has been around for 60 years, and, if implemented properly, it is still the fastest option for many sorting tasks. According to the description on Wikipedia, a well designed Quicksort is "…somewhat faster than Merge sort and about two or three times faster than Heapsort."
Many Linux users today have studied Quicksort at some point in the past, through a computer science class or other training scenario, but unless you are working as a professional programmer, chances are it has been a few years since you have taken the time to ponder the elegant Quicksort algorithm. Still, sorting goes on all the time on Linux networks. You don't have to be a full-time app developer to conjure up an occasional script to rank results or order a set of values extracted from a log file. This article explores some of the nuances of the classic Quicksort.
Quicksort ABC
The Quicksort [1] algorithm originated with Tony Hoare [2], who first developed it in 1959 and published it in 1961. Quicksort is what is known as a divide-and-conquer algorithm. One element in the array is chosen to be the pivot element. All elements smaller than the pivot element are then grouped in a sub-array before it, and all elements larger than the pivot element are placed in a sub-array after it. This process is then repeated with the sub-arrays: a pivot element is chosen, with smaller elements placed in a sub-array before and larger elements placed in a sub-array after. After a finite number of steps, the size of the sub-arrays becomes one, and at that point, the whole array has been sorted.
Too complicated? Figure 1 sums up the Quicksort algorithm. Boxes containing only one red number are already in the right position. In the first row, the number 6 is the pivot element. Now all of the elements are sorted in relation to 6. In the second row, the 5 and the 9 act as the new pivot elements. Now the partial arrays are sorted relative to 5 and 9. The result is the third row, where almost all of the elements are already sorted. Only the 8 (the new pivot element) has to swap places with the 7. If you would prefer an animated clip of the Quicksort algorithm, check out the Quicksort page on Wikipedia [1].
If the array contains n elements, an average of n*log(n) sorting steps are needed. The log(n) factor results from the fact that the algorithm halves the array in each step. In a worst-case scenario, Quicksort requires n*n sorting steps. To illustrate this worst-case scenario, consider that, in Figure 1, the middle element served as the pivot element. But any other element could also serve as the pivot element. If the first element is the pivot element and the array is already sorted in ascending order, the array must be halved exactly n times, which would be the (very unlikely) worst case. Note that a few lines of text are all I needed to describe the elegant and highly efficient Quicksort algorithm, along with its performance characteristics.
First Encounter
The classic Quicksort implementation in C lacks charm and is quite successful at disguising its elegant design, as Listing 1 demonstrates. I won't provide a full description of the code; however, one observation is very interesting.
Listing 1
Quicksort in C
01 void quickSort(int arr[], int left, int right) { 02 int i = left, j = right; 03 int tmp; 04 int pivot = arr[abs((left + right) / 2)]; 05 while (i <= j) { 06 while (arr[i] < pivot) i++; 07 while (arr[j] > pivot) j--; 08 if (i <= j) { 09 tmp = arr[i]; 10 arr[i] = arr[j]; 11 arr[j] = tmp; 12 i++; j--; 13 } 14 } 15 if (left < j) quickSort(arr, left, j); 16 if (i < right) quickSort(arr, i, right); 17 }
In Lines 9 to 11, the code overwrites the existing elements. Thus, the algorithm runs in-place and assumes mutable data. A nice expression has been established for the task of overwriting old values with new ones in functional programming: destructive assignment [3]. This takes us neatly to the next topic: in functional programming languages like Haskell, Quicksort is represented in a far more elegant way.
Second Encounter
In Haskell, data is immutable, which precludes destructive assignment by design. The Haskell-based Quicksort algorithm in Listing 2 creates a new list in each iteration, rather than acting directly on the array as in Listing 1. Quicksort in two lines? Is that all there is to it? Yes.
Listing 2
Quicksort in Haskell
qsort [] = [] qsort (x:xs) = qsort [y | y <- xs, y < x] ++ [x] ++ qsort [y | y <- xs, y >= x]
The qsort
algorithm consists of two function definitions. The first line applies the defined Quicksort to the empty list. The second line represents the general case, where the list consists of at least one element: x:xs
. Here, by convention, x
denotes the beginning of the list and xs
denotes the remainder.
The strategy of the Quicksort algorithm can be implemented almost directly in Haskell:
- use the first element of the list
x
as the pivot element; - insert (
(++)
) all elements inxs
that are lesser thanx
((qsort [y | y <- xs, y < x])
) in front of the one-element list[x]
; - append all elements in
xs
that are at least as large asx
to the list[x]
((qsort [y | y <- xs, y >= x])
).
The recursion ends when Quicksort is applied to the empty list. Admittedly, the compactness of Haskell seems unusual. However, this Quicksort algorithm can be implemented in any programming language that supports list comprehension – which leads to the more mainstream Python programming language in Listing 3.
Listing 3
Quicksort in Python
def qsort(L): if len(L) <= 1: return L return qsort([lt for lt in L[1:] if lt < L[0]]) + L[0:1] + qsort([ge for ge in L[1:] if ge >= L[0]])
The description of the Haskell algorithm can be applied almost verbatim to Python. The subtle difference is that in Python, L[0:1]
acts as the first element and you express the list concatenation in Python with the +
symbol. The algorithm reliably performs its services in Figure 2.
Peaceful Combination
Admittedly, both implementation strategies for Quicksort have their advantages and disadvantages. The imperative strategy in Listing 1 requires no additional memory but almost completely obscures the elegant structure of the algorithm. The functional strategy in Listing 2 and Listing 3 looks very elegant but creates a new list with each recursion. Is it possible to combine the advantages of both worlds?
Yes, it is. The following implementation in C++ is based on std::partition
[4], an algorithm from the Standard Template Library that partitions a range based on an element. std::partition
in Listing 4 does the job of list comprehension in Listing 2 and Listing 3.
Listing 4
Quicksort in C++
template <typename Forward>; void quicksort(ForwardIt first, ForwardIt last) { if(first == last) return; auto pivot = *std::next(first, std::distance(first,last)/2); ForwardIt middle1 = std::partition(first, last, [pivot](const auto& em){return em < pivot;}); ForwardIt middle2 = std::partition(middle1, last, [pivot](const auto& em){return !(pivot < em);}); quicksort(first, middle1); quicksort(middle2, last); }
The Crucial Question
But this article has not yet answered the crucial question in C++: "Say, how fast are you?"
In C++17, C++ introduced support for the Parallel Standard Template Library (STL). Around 70 of the more than 100 algorithms in the STL can be executed sequentially, executed in parallel, or vectorized by means of a policy. However, so far only the Microsoft compiler supports parallel execution of the STL algorithms, which is why the following performance values are based on the MS compiler. All performance figures are taken from a program compiled for maximum optimization and executed with eight cores. Listing 5 uses a slightly modified variant of the Quicksort algorithm from Listing 4.
Listing 5
Performance Test
01 #include <algorithm> 02 #include <chrono> 03 #include <execution> 04 #include <iomanip> 05 #include <iostream> 06 #include <iterator> 07 #include <numeric> 08 #include <random> 09 #include <vector> 10 11 template <typename ExecutionPolicy, typename ForwardIt> 12 void quicksort(ExecutionPolicy&& policy, ForwardIt first, ForwardIt last) { 13 if(first == last) return; 14 auto pivot = *std::next(first, std::distance(first,last)/2); 15 ForwardIt middle1 = std::partition(policy, first, last, [pivot](const auto& em){ return em < pivot; }); 16 ForwardIt middle2 = std::partition(policy, middle1, last, [pivot](const auto& em){ return !(pivot < em); }); 17 quicksort(policy, first, middle1); 18 quicksort(policy, middle2, last); 19 } 20 21 std::vector<int> getRandomVector(int numberRandom, int lastNumber) { 22 std::random_device seed; 23 std::mt19937 engine(seed()); 24 std::uniform_int_distribution<int> dist(0, lastNumber); 25 std::vector<int> randNumbers; 26 randNumbers.reserve(numberRandom); 27 for 28 (int i=0; i < numberRandom; ++i){ 29 randNumbers.push_back(dist(engine)); 30 } 31 return randNumbers; 32 } 33 34 int main() { 35 36 std::cout << :*\n:*; 37 38 constexpr int numberRandom = 100:*000:*000; 39 constexpr int lastNumber = 100; 40 41 std::vector randVec = getRandomVector(numberRandom, lastNumber); 42 43 std::cout << numberRandom << " random numbers between 0 and " << lastNumber << "\n\n"; 44 45 { 46 std::vector tmpVec = randVec; 47 std::cout << std::fixed << std::setprecision(10); 48 auto begin = std::chrono::steady_clock::now(); 49 quicksort(std::execution::seq, tmpVec.begin(), tmpVec.end()); 50 std::chrono::duration<double> last= std::chrono::steady_clock::now() - begin; 51 std::cout << "Quicksort sequential: " << last.count() << :*\n:*; 52 } 53 54 { 55 std::vector tmpVec = randVec; 56 std::cout << std::fixed << std::setprecision(10); 57 auto begin = std::chrono::steady_clock::now(); 58 quicksort(std::execution::par, tmpVec.begin(), tmpVec.end()); 59 std::chrono::duration<double> last= std::chrono::steady_clock::now() - begin; 60 std::cout << "Quicksort parallel: " << last.count() << :*\n:*; 61 } 62 63 std::cout << "\n\n"; 64 65 { 66 std::vector tmpVec = randVec; 67 std::cout << std::fixed << std::setprecision(10); 68 auto begin = std::chrono::steady_clock::now(); 69 std::sort(std::execution::seq, tmpVec.begin(), tmpVec.end()); 70 std::chrono::duration<double> last= std::chrono::steady_clock::now() - begin; 71 std::cout << "std::sort sequential: " << last.count() << :*\n:*; 72 } 73 74 { 75 std::vector tmpVec = randVec; 76 std::cout << std::fixed << std::setprecision(10); 77 auto begin = std::chrono::steady_clock::now(); 78 std::sort(std::execution::par, tmpVec.begin(), tmpVec.end()); 79 std::chrono::duration<double> last= std::chrono::steady_clock::now() - begin; 80 std::cout << "std::sort parallel: " << last.count() << :*\n:*; 81 } 82 83 std::cout << :*\n:*; 84 }
The algorithm in Listing 5 includes an execution strategy (Line 12). This lets you control whether the algorithm is called sequentially (std::execution::seq
in Lines 49 and 69) or in parallel (std::execution::par
in Lines 58 and 78). Put simply, the program sorts a vector of 100,000,000 random elements (Line 38) between 0 and 100 (Line 39) and measures the performance four times.
The task of generating the equally distributed random numbers is handled by the getRandom
function in Line 21. The vector is sorted both sequentially (Lines 45 to 52) and in parallel (Lines 54 to 61) with the quicksort
function and sequentially (Lines 65 to 72) and in parallel (lines 74 to 81) with std::sort
from the STL. In doing so, std::sort
applies a hybrid method under the hood that also uses Quicksort. If you are interested in the details of Microsoft's implementation of std::sort
, you can check them out on GitHub [5].
In addition to the execution strategy, two other C++17 features are used in this example. On the one hand, numbers can be represented in a readable way (100'000'000
) with ticks; on the other, the compiler can automatically determine the type of a class template: std::vector tmpVec = randVec
instead of std::vector<int> tmpVec = randVec
.
Size Matters
But now back to the crucial question. Given 100,000,000 random numbers in the range of 0 to 100, the Quicksort executed in parallel is impressively fast (Figure 3). It beats the sequential variant by a factor of 5 and is more than twice as fast as the std::sort
executed in parallel. However, this relation changes significantly if the range from which the random numbers are taken in a uniform distribution grows significantly.
Regardless of whether the range increases to 0 to 10,000 (Figure 4) or 0 to 1,000,000 (Figure 5), the std::sort
executed in parallel beats the sequentially-executed version by a factor of about 4. But the superior performance of parallel execution dwindles significantly as the range increases. For random numbers between 0 and 1,000,000, parallel execution is slower than sequential execution by a factor of 5.
What can these serious differences in performance be attributed to? We will not be analyzing the problem exhaustively, but only looking to provide some food for thought.
The main difference between 100,000,000 random numbers between 0 and 100 and between 0 and 1,000,000 is that, in the first case, on average, 1,000,000 equal numbers (1,000,000/100) and in the second case, on average, 100 equal numbers (100,000,000 / 1,000,000) end up in the random vector. In particular, this means that the call to std::partition
[4] is far more efficient in the first case, since it has to swap significantly fewer elements relative to the pivot element. Moreover, this write access requires synchronization between threads.
Now I have to get personal. During my long time as a C++ software developer and now as a C++ trainer, I have become prejudiced: "Don't think you can beat the performance of STL algorithms in general." I almost gave up on my fixed opinion after the first run with the random numbers between 0 and 100; however, the further performance tests just reinforced it.
Conclusions
Is Quicksort my favorite algorithm? I am not sure. In any case, it is clear that an understanding of this algorithm is part of the mandatory repertoire of every professional software developer. Annoyingly, the classical implementation of the Quicksort algorithm obscures its elegant structure and thus also complicates its basic understanding.
Infos
- Quicksort: https://en.wikipedia.org/wiki/Quicksort
- Tony Hoare: https://en.wikipedia.org/wiki/Tony_Hoare
- Destructive Assignment: https://en.wikipedia.org/wiki/Assignment_(computer_science)
- std::partition: https://en.cppreference.com/w/cpp/algorithm/partition
- Microsoft's STL implementation: https://github.com/microsoft/STL