Getting started with the R data analysis language
Number Game
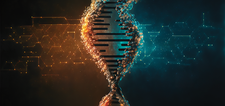
The R programming language is a universal tool for data analysis and machine learning.
The R language is one of the best solutions for statistical data analysis. R is ideal for tasks such as data science and machine learning. R, which was created by Ross Ihaka and Robert Gentleman at the University of Auckland in 1991, is a GNU project that is similar to the S language, which was developed in the 1970s at Bell Labs.
R is an interpreted language. Input is either executed directly in the command-line interface or collected in scripts. The R language is open source and completely free. R, which runs on Linux, Windows, and macOS, has a large and active community that is constantly creating new, customized modules.
R was developed for statistics, and it comes with fast algorithms that let users analyze large datasets. There is a free and very well-integrated development environment named RStudio, as well as an excellent help system that is available in many languages.
[...]