Indoor navigation with machine learning
GPS lets you determine the position of an object down to single-centimeter accuracy – as long as the object is outside. If the object is inside, the task is a bit more complicated. Satellite navigation doesn't work well through doors and rooftops, and even if you could replace the satellite signal with equivalent transmissions from locally placed beacons or WLAN access points, the presence of interior walls and furniture muddles up the results of classical analytical techniques such as those used with GPS. What is more, when someone is inside a building, the question is not so much about "What are his coordinates." What you really want to know is "What room is he in?" Such a problem is better addressed through the tools of machine learning.
Of course, creating a complete machine learning solution to find someone in a small house might seem like overkill, but this article is intended as an exercise to show these machine learning tools and techniques in a simple situation – a kind of machine learning "Hello, World" application. One could imagine scenarios where these techniques could find broader utility, such as tracking down an executive in a large office complex or even finding a lost set of car keys.
In this example, Tom, the protagonist, has lost his way. Fortunately, his smartphone shows the signal strength of seven hotspots in his vicinity (Figure 1). Because Tom often gets lost, I have mapped the four rooms as a precaution (the blue crosses in Figure 2), and I have a machine learning dataset I can use to train a program to find Tom.
[...]
Buy this article as PDF
(incl. VAT)
Buy Linux Magazine
Subscribe to our Linux Newsletters
Find Linux and Open Source Jobs
Subscribe to our ADMIN Newsletters
Support Our Work
Linux Magazine content is made possible with support from readers like you. Please consider contributing when you’ve found an article to be beneficial.
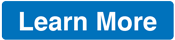
News
-
CachyOS Now Lets Users Choose Their Shell
Imagine getting the opportunity to select which shell you want during the installation of your favorite Linux distribution. That's now a thing.
-
Wayland 1.24 Released with Fixes and New Features
Wayland continues to move forward, while X11 slowly vanishes into the shadows, and the latest release includes plenty of improvements.
-
Bugs Found in sudo
Two critical flaws allow users to gain access to root privileges.
-
Fedora Continues 32-Bit Support
In a move that should come as a relief to some portions of the Linux community, Fedora will continue supporting 32-bit architecture.
-
Linux Kernel 6.17 Drops bcachefs
After a clash over some late fixes and disagreements between bcachefs's lead developer and Linus Torvalds, bachefs is out.
-
ONLYOFFICE v9 Embraces AI
Like nearly all office suites on the market (except LibreOffice), ONLYOFFICE has decided to go the AI route.
-
Two Local Privilege Escalation Flaws Discovered in Linux
Qualys researchers have discovered two local privilege escalation vulnerabilities that allow hackers to gain root privileges on major Linux distributions.
-
New TUXEDO InfinityBook Pro Powered by AMD Ryzen AI 300
The TUXEDO InfinityBook Pro 14 Gen10 offers serious power that is ready for your business, development, or entertainment needs.
-
LibreOffice Tested as Possible Office 365 Alternative
Another major organization has decided to test the possibility of migrating from Microsoft's Office 365 to LibreOffice.
-
Linux Mint 20 Reaches EOL
With Linux Mint 20 at its end of life, the time has arrived to upgrade to Linux Mint 22.